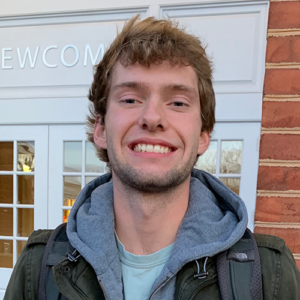
Background
University of Virginia, Class of 2021
Project
Ultrasound Microbubble Tumor Analysis
Project Overview
The goal of this project was to use microbubble intensity data over time to classify tumors from normal brain tissue and arteries. To do this, the data was preprocessed, engineered, and analyzed using machine learning techniques.
Project Outcomes
Although there is still much work to be done, we were able to find that unsupervised learning (clustering) methods outperform supervised learning methods when classifying the data. Furthermore, after tweaking our methods based on different patients, we obtained a high accuracy of clustering (~90% or above) when using ~100 regions of interest (ROI). This is an encouraging start, as unsupervised methods do not require previous knowledge of each ROI’s label (tumor, artery, etc.).
Describe your experience as a Foundation intern.
My experience as an intern was different than others, as I worked on a collaboration project strictly via Zoom. However, I thoroughly enjoyed every moment of it! Working on ultrasound-based research, learning new techniques and skills, and being guided by two supervisors who are respected in their fields was an extraordinary opportunity that has given me the desire to continue to do research outside of college.
How will your accomplishments impact the field of focused ultrasound?My hope is that my accomplishments will show the focused ultrasound community how machine learning can be a powerful method for classifying ultrasound data, classifying different tumor types, and allowing doctors to use focused ultrasound more effectively when imaging tumors.
Has your internship affected your career plans? If so, how?
Yes, because of my experience as an intern I plan to work as a Research Assistant for Boston Children’s Hospital for a few years with a goal of applying to graduate school to continue to do Biostatistical research!